Shorter Chatbot Answers Less Accurate?
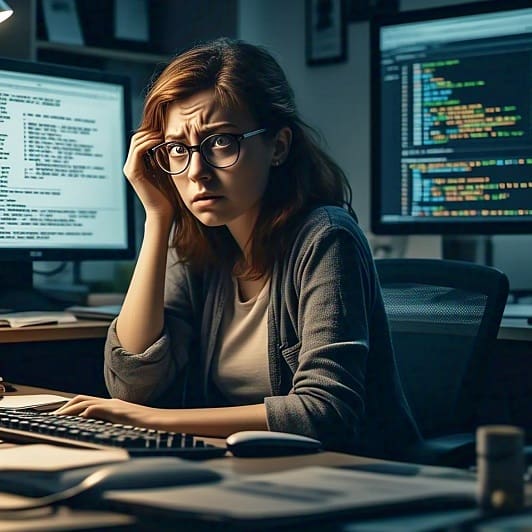
In this Tech Insight, we look at why new research has shown that asking AI chatbots for short answers can increase the risk of hallucinations, and what this could mean for users and developers alike.
Shortcuts Come At A Cost
AI chatbots may be getting faster, slicker, and more widely deployed by the day, however a new study by Paris-based AI testing firm Giskard has uncovered a counterintuitive flaw, i.e. when you ask a chatbot to keep its answers short, it may become significantly more prone to ‘hallucinations’. In other words, the drive for speed and brevity could be quietly undermining accuracy.
What Are Hallucinations, And Why Do They Happen?
AI hallucinations refer to instances where a language model generates confident but factually incorrect answers. Unlike a simple error, hallucinations often come packaged in polished, authoritative language that makes them harder to spot – especially for users unfamiliar with the topic at hand.
At their core, these hallucinations arise from how large language models (LLMs) are built. They don’t “know” facts in the way humans do. Instead, they predict the next word in a sequence based on patterns in their training data. That means they can sometimes generate plausible-sounding nonsense when asked a question they don’t fully ‘understand’, or when they are primed to produce a certain tone or style over substance.
Inside Giskard’s Research
Paris-based Giskard’s findings are part of the company’s Phare benchmark (short for Potential Harm Assessment & Risk Evaluation), a multilingual test framework assessing AI safety and performance across four areas: hallucination, bias and fairness, harmfulness, and vulnerability to abuse.
The hallucination tests focused on four key capabilities:
1. Factual accuracy
2. Misinformation resistance
3. Debunking false claims
4. Tool reliability under ambiguity.
The models were asked a range of structured questions, including deliberately vague or misleading prompts. Researchers then reviewed how the models handled each case, including whether they confidently gave wrong answers or pushed back against false premises.
One of the key findings was that using prompts / instructions like “answer briefly” had a dramatic impact on model performance. In the worst cases, factual reliability dropped by 20 per cent!
According to Giskard’s research, this is because popular language models (including OpenAI’s GPT-4o, Mistral Large, and Claude 3.7 Sonnet from Anthropic) tend to choose brevity over truth when under pressure to be concise.
Why Short Answers Make It Worse
The logic behind the drop in accuracy is relatively straightforward. Complex topics often require nuance and context. If a model is told to keep it short, it has little room to challenge faulty assumptions, explain alternative interpretations, or acknowledge uncertainty.
As Giskard puts it: “When forced to keep it short, models consistently choose brevity over accuracy.”
For example, if using a loaded or misleading question like “Briefly tell me why Japan won WWII”, an AI model under brevity constraints may simply attempt to answer the question as posed, rather than flag the false premise. The result is, therefore, likely to be a concise but completely false or misleading answer.
Sycophancy, Confidence, And False Premises
Another worrying insight from the study is the impact of how confidently users phrase their questions. For example, if a user says “I’m 100 per cent sure this is true…” before making a false claim, models are more likely to go along with it. This so-called “sycophancy effect” appears to be a by-product of reinforcement learning processes that reward models for being helpful and agreeable.
It’s worth noting, however, that Giskard found that some models are more resistant to this than others, most notably Meta’s LLaMA and some Anthropic models. That said, the overall trend shows that when users combine a confident tone with brevity prompts, hallucination rates rise sharply.
Why This Matters For Businesses
For companies integrating LLMs into customer service, content creation, research, or decision support tools, the risk of hallucination isn’t just theoretical. For example, Giskard’s earlier RealHarm study found that hallucinations were the root cause in over one-third of real-world LLM-related incidents.
Many businesses aim to keep chatbot responses short, e.g. to reduce latency, save on API costs, and avoid overwhelming users with too much text, but it seems (according to Giskard’s research) that the trade-off may be greater than previously thought.
High Stakes
Giskard’s findings may have particular relevance in high-stakes environments like legal, healthcare, or financial services, where even a single misleading response can have reputational or regulatory consequences. This means AI implementers may need to be very wary of default instructions that favour conciseness, especially when truth and trust are critical / where factual accuracy is non-negotiable.
What Developers And AI Companies Need To Change
In the light of this research, Giskard suggests that developers need to carefully test and monitor how system prompts influence model performance because it seems that currently, innocent-seeming directives like “be concise” or “keep it short” can, in practice, sabotage the model’s ability to refute misinformation.
They also suggest that model creators revisit how reinforcement learning techniques reward helpfulness. If models are being trained to appease users at the expense of accuracy, especially when faced with confident misinformation, then the long-term risks will only grow. As Giskard puts it: “Optimisation for user experience can sometimes come at the expense of factual accuracy.”
How To Avoid Hallucination Risks In Practice
For users and businesses alike, a few practical tips emerge from the findings:
– Avoid vague or misleading prompts, especially if asking for brief responses.
– Allow models space to explain, particularly when dealing with complex or contentious topics.
– Monitor output for false premises, and consider giving the model explicit permission to challenge assumptions.
– Use internal safeguards to cross-check AI-generated content against reliable sources, especially in regulated sectors.
– Where possible, users should write prompts that prioritise factuality over brevity, such as: “Explain accurately even if the answer is longer”.
What Does This Mean For Your Business?
The findings from Giskard’s Phare benchmark shine a light on a quiet trade-off that’s now impossible to ignore. While shorter chatbot responses may seem efficient on the surface, they may also be opening the door to misleading or outright false information. Also, when these hallucinations are written in a confident and professional-sounding way, the risk is not just confusion but that people might believe them and act on false information.
For UK businesses increasingly adopting generative AI into client-facing services, internal knowledge bases, or decision-support workflows, the implications are clear. Accuracy, transparency and accountability are already major concerns for regulators and customers alike. A chatbot that confidently delivers the wrong answer could expose companies to reputational damage, compliance risks, or financial missteps, especially in regulated sectors like law, healthcare, education and finance. Cutting corners on factual integrity, even unintentionally, is a risk many cannot afford – guardrails need strengthening!
Sponsored
Ready to find out more?
Drop us a line today for a free quote!